Velocity Model Building From Raw Shot Gathers Using Machine Learning Feature, & More
Introduction
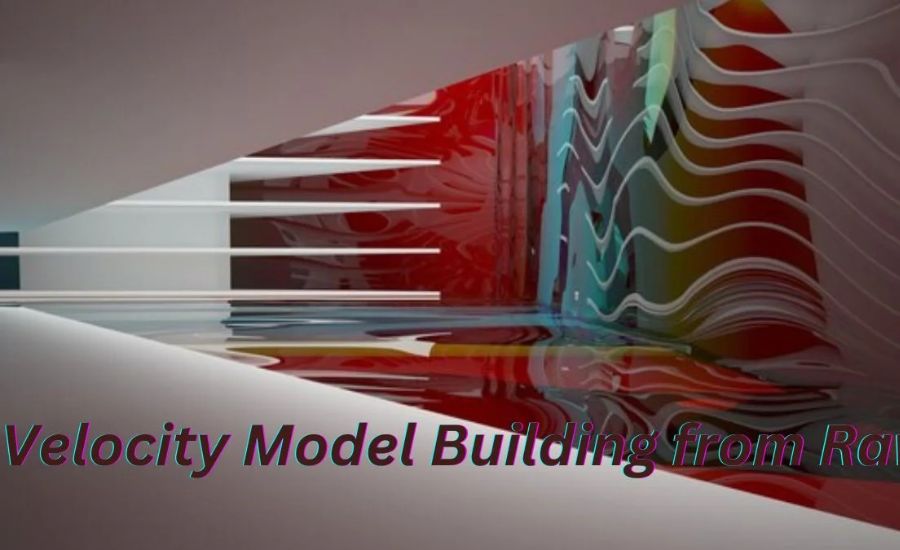
Speed models are fundamental in seismic investigation as they assume a vital part in imaging and deciphering the World’s subsurface. These models show how seismic waves travel through various layers of the Earth, which is critical for identifying and examining subsurface elements like oil and gas repositories. Customary techniques for making speed models can be very mind boggling, including iterative cycles that are both tedious and computationally demanding. These customary methodologies frequently rely upon manual translation, which can present blunders and emotional inclinations.In this article we’ll read about Velocity Model Building From Raw Shot Gathers Using Machine Learning in detail.
What is Velocity Model Building?
Speed model structure includes making a point by point portrayal of how seismic waves travel through the World’s subsurface. This cycle is basic for precisely deciphering seismic information, which assists in recognizing subsurface designs and finding important assets with preferring oil, gas, and minerals.
Generally, building these models includes breaking down information from seismic overviews. This incorporates analyzing crude shot accumulates, which are accounts of seismic waves bouncing off subsurface designs. Fostering a speed model from these accounts is fundamental for different applications, including investigating normal assets, directing ecological examinations, and performing geotechnical examinations.
Why Machine Learning is Ideal for Velocity Model Building
Applying AI to speed model structure offers a few particular advantages. One significant benefit is the speed at which AI models can handle immense measures of information. Conventional techniques frequently battle with the enormous volumes of information delivered by seismic studies, however AI can deal with this proficiently, which is particularly valuable for continuous information handling during investigation.
AI likewise sparkles while managing perplexing and loud datasets. Seismic information can be tried because of covering signals and intrinsic commotion. While customary techniques could find it challenging to isolate these signs precisely, AI calculations, especially those using profound learning, can successfully distinguish and separate significant elements with high accuracy.
Furthermore, AI models improve with experience. As additional seismic information is gathered and used to prepare these models, their precision upgrades after some time. This continuous refinement is essential in speed model structure, where even minor mistakes can prompt critical errors in subsurface imaging.
How Machine Learning Transforms Velocity Model Building
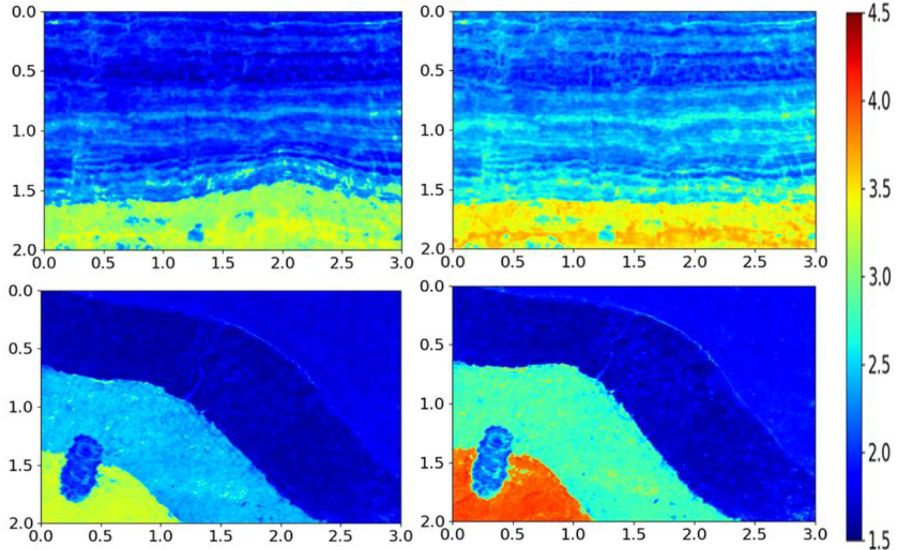
AI is altering speed model structure via mechanizing and refining the cycle in more than one way:
1. Automated Feature Extraction
AI calculations are profoundly viable at robotizing the extraction of highlights from seismic shot assemblies. Dissimilar to customary strategies that depend on manual translation, these calculations can naturally recognize and take out significant elements from crude information with noteworthy exactness. This mechanization speeds up the interaction as well as decreases the gamble of human blunder.
2. Advanced Pattern Recognition
AI, especially with profound learning strategies, succeeds at perceiving unpredictable examples inside seismic information. These models can uncover unobtrusive connections and highlights that customary techniques could disregard, prompting more exact speed models.
3. Predictive Modeling
Via preparing on broad authentic seismic information, AI calculations can anticipate speed models with high exactness. This prescient capacity considers more dependable and definite speed models by utilizing past information to conjecture future outcomes.
4. Handling Large Datasets
Seismic studies produce huge measures of information, which can be overpowering for conventional handling techniques. AI calculations are explicitly intended to oversee and investigate enormous datasets proficiently, making them appropriate for current seismic investigation, where information volumes keep on developing.
Feature Engineering from Shot Gathers
In seismic information handling, highlight designing includes separating and dissecting different traits from seismic shot accumulates, like recurrence, stage, or envelope plentifulness. These traits are fundamental as they offer important experiences into the subsurface and help AI models in separating between different topographical highlights.
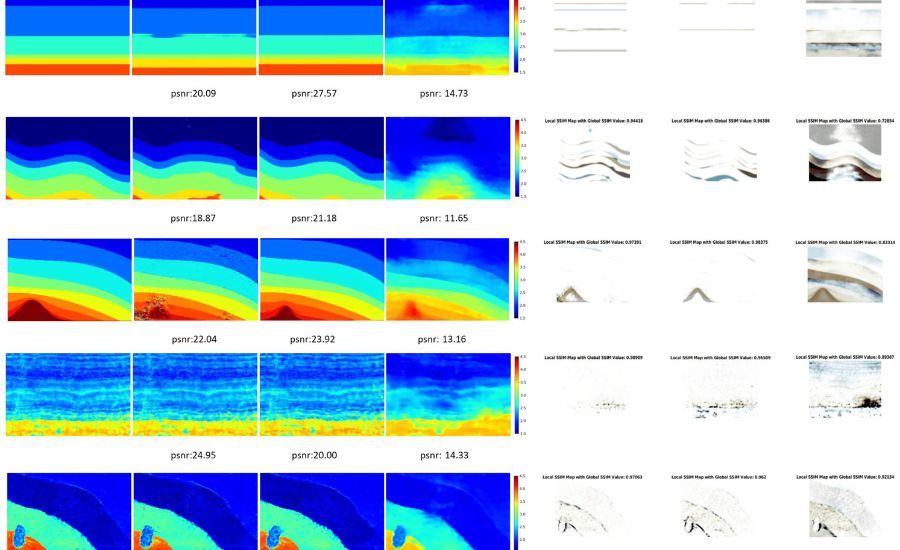
Moreover, dimensionality decrease strategies, similar to Head Part Examination (PCA), are utilized to improve on the information while holding key examples. This approach is basic for improving AI models, especially while dealing with broad seismic datasets. By decreasing the information’s intricacy, PCA assists the models with performing all the more productively and precisely.
Benefits of Machine Learning in Velocity Model Building
1. Enhanced Accuracy
AI models offer prevalent exactness in foreseeing speed models contrasted with customary procedures. By uncovering complex examples in seismic information, these calculations convey more exact outcomes, which are critical for dependable subsurface examination.
2. Increased Efficiency
The computerization capacities of AI decisively speed up the speed model structure process. This lift in proficiency works with speedier navigation and abbreviates the time expected to finish seismic investigation projects.
3. Reduced Human Error
AI essentially decreases the gamble of human blunder, which is normal in manual techniques. The information driven nature of ML models guarantees reliable and trustworthy outcomes, working on the general nature of the speed model.
4. Scalability
AI calculations are intended to deal with huge datasets successfully, making them appropriate for the developing volumes of seismic information. Their adaptability empowers intensive examination and exact model structure, even as information sizes increase.
Case Studies and Real-World Applications
Case Study 1: Oil and Gas Exploration
An oil and gas investigation firm as of late embraced AI to improve their speed model structure. By incorporating ML calculations, they accomplished a quicker and more exact examination of seismic information. This progression brought about a more exact speed model, which fundamentally worked on their capacity to pinpoint promising boring locales. Subsequently, the organization saw an expansion in fruitful investigation results and an eminent decrease in penetrating expenses.
Case Study 2: Mineral Exploration
A mining organization carried out AI to refine their seismic overviews for mineral investigation. The utilization of ML models empowered them to handle enormous datasets all the more productively, which further developed their asset assessment and considered more designated penetrating. The utilization of AI decreased investigation gambles as well as upgraded their asset the executives techniques.
Traditional Methods of Velocity Model Building
Before the appearance of AI, speed model structure depended intensely on manual and iterative strategies. These conventional strategies, albeit compelling, were tedious and powerless to human mistake. They likewise confronted hardships in scaling to oblige the enormous datasets ordinary of present day seismic studies.
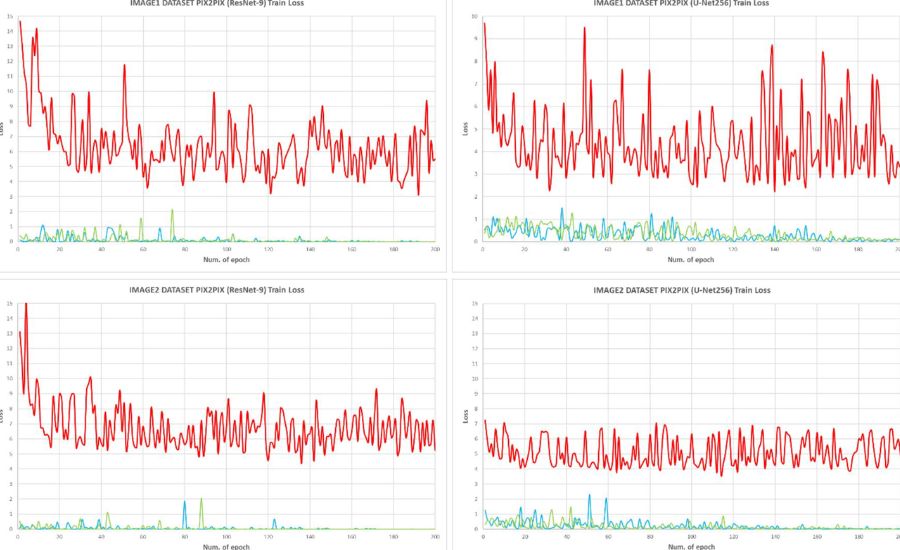
The essential impediments of these conventional methodologies remember their dependence for master understanding, the intricacy of dealing with huge volumes of information, and the high computational requests of over and over reproducing seismic wave spread.
Challenges in Velocity Model Building
Precisely developing speed models from seismic information presents a few difficulties. One significant issue is the intrinsic commotion in the information, which requires broad preprocessing to guarantee lucidity and unwavering quality. Furthermore, the reversal cycle, used to get speed gauges from seismic information, can be both computationally requesting and delicate to information varieties, bringing about likely mistakes in the speed model.
One more huge test is the subjectivity associated with manual translation. Various specialists could break down similar information in an unexpected way, prompting conflicting speed models. This changeability, combined with the intricacy and volume of seismic information, has prompted developing interest in mechanizing the cycle with AI to improve precision and proficiency.
Types of Machine Learning Used in Seismic Data
A few kinds of AI strategies are important for seismic information handling, each offering novel benefits:
Supervised learning, Directed learning includes preparing models utilizing named information, where the results are known. With regards to seismic information, this implies utilizing verifiable seismic information with realized speed models to prepare calculations. When prepared, these models can anticipate speed models for new, concealed seismic shot assemblies. This approach is especially viable when there is an abundance of marked information accessible.
Unsupervised learningUnaided learning, conversely, doesn’t depend on named information. All things considered, it recognizes examples and designs inside the information without anyone else. For seismic information handling, solo learning can be utilized to group seismic information into various areas in light of likenesses. This can be helpful for distinguishing and ordering topographical highlights without earlier information on their precise qualities.
Reinforcement learning
Support learning is an arising region inside AI where a specialist figures out how to pursue choices through collaborations with its current circumstance and getting input. In geophysical applications, this technique includes preparing models to streamline choices in light of remunerations or punishments. For seismic information handling, support learning can be utilized to refine methodologies for information obtaining and translation, working on generally proficiency and precision.
Steps in Velocity Model Building Using Machine Learning
Building a speed model with AI includes a few pivotal moves toward guaranteeing the last model is both exact and dependable. Here is an outline of the cycle:
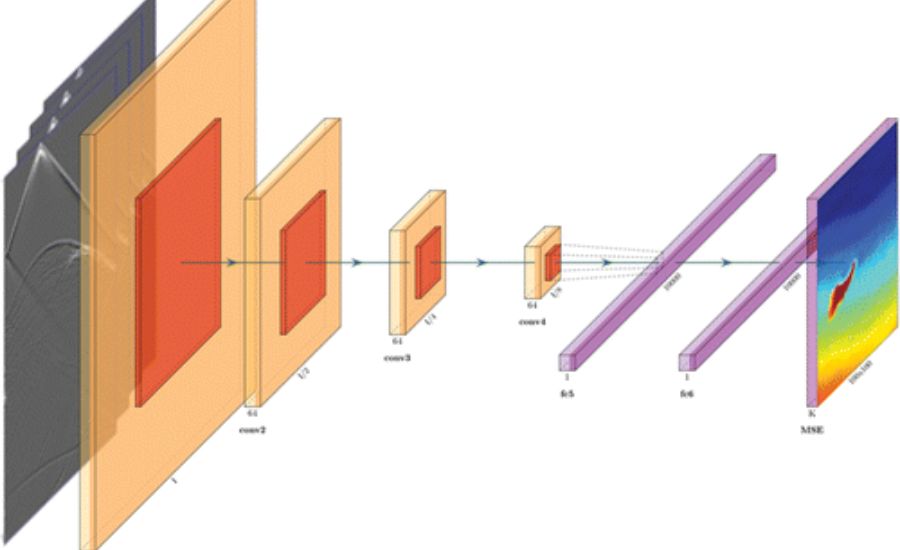
Data Acquisition:The initial step is to accumulate crude shot accumulates from seismic reviews, which act as the contribution for the AI model. The nature of this information is central, as the model’s viability is vigorously subject to the precision and uprightness of the information.
Data Preprocessing:
Seismic information, like crude shot accumulates, frequently contain commotion and need preprocessing to be helpful for AI. This step includes a few undertakings, including sound decrease, information standardization, and component extraction. The objective of preprocessing is to change the crude information into a configuration that the AI calculations can process really.
Model Selection: Choosing the proper AI model is basic to the progress of the speed model structure process. Contingent upon the qualities of the information and the undertaker’s particular requirements, different calculations might be thought of, for example, administered learning models, brain organizations, or profound learning procedures.
Training the Model:
In the wake of choosing a model and setting up the information, the following stage is to prepare the model. This includes taking care of it with a subset of the preprocessed shot assemblies, permitting it to learn designs inside the information. In the interim, the model’s exhibition is constantly evaluated and changed in accordance with further development.
Validation and Testing:
When the model is prepared, it should be approved and tried with a different dataset to guarantee its power and capacity to sum up to new, inconspicuous information. This step confirms that the model isn’t only retaining the preparation information however is really learning the hidden examples.
Deployment:
The last step includes conveying the prepared model in a certifiable situation. This implies coordinating the model into the seismic information handling work process, empowering it to create speed models from new seismic information progressively, accordingly upgrading the general proficiency and precision of seismic investigation.
Future Prospects
The fate of speed model structure utilizing AI seems, by all accounts, to be profoundly encouraging. With continuous mechanical headways, AI calculations are expected to turn out to be progressively complex, prompting more prominent precision and proficiency in making speed models. Besides, the joining of AI with arising advances like distributed computing and high level information examination is supposed to further upgrade its capacities, giving useful assets to seismic investigation and working on generally speaking results in the field.
Labeling in Machine Learning for Velocity Models
In regulated learning, one of the most basic errands is naming the preparation information precisely. For seismic speed models, this frequently includes giving the right speed model relating to each shot assembled in the preparation dataset. Notwithstanding, this interaction can be very challenging, as it for the most part requires either manual translation, which is tedious and inclined to blunders, or the utilization of manufactured information.
A typical way to deal with making these marks is to utilize forward demonstrating strategies to create engineered shot accumulates, where the speed models are now known. This technique considers the production of named datasets that can be utilized to prepare AI calculations.
Facts:
Velocity Models in Seismic Exploration: Velocity models are crucial for seismic exploration, helping interpret subsurface features such as oil and gas reservoirs by illustrating how seismic waves travel through different Earth layers.
- Traditional Methods: Conventional velocity model-building methods are manual, iterative, and time-consuming, relying heavily on expert interpretation, which introduces potential human errors and biases.
- Machine Learning Advantages: Machine learning (ML) offers several advantages over traditional methods, including improved accuracy, increased efficiency, reduced human error, and scalability to handle large datasets.
- Types of Machine Learning: Different ML approaches—such as supervised learning, unsupervised learning, and reinforcement learning—are utilized in seismic data processing to enhance velocity model building.
- Feature Engineering: In seismic data processing, feature engineering involves extracting key attributes (e.g., frequency, phase, amplitude) from shot gathers, which are essential for ML models to distinguish between different geological features.
- Future Prospects: The future of velocity model building with ML looks promising, with advancements in technology and integration with cloud computing and advanced analytics expected to further enhance capabilities.
- Case Studies: Real-world applications in the oil and gas industry and mineral exploration demonstrate how ML models have improved the accuracy of velocity models, leading to better drilling decisions and reduced costs.
Summary :
The article discusses the importance of velocity models in seismic exploration, highlighting their role in identifying subsurface features such as oil, gas, and mineral deposits. Traditional methods of velocity model building are described as labor-intensive, error-prone, and inefficient, especially when dealing with large datasets typical of modern seismic surveys. The article introduces machine learning as a powerful alternative, capable of automating the velocity model-building process while enhancing accuracy, reducing human error, and improving scalability. It outlines various machine learning techniques applicable in this domain, such as supervised learning for predictive modeling, unsupervised learning for clustering, and reinforcement learning for decision-making. The article also provides case studies from the oil and gas and mining industries, demonstrating the practical benefits of ML in seismic data processing. The piece concludes with an optimistic outlook on the future of ML in velocity model building, emphasizing continued advancements in technology and data processing capabilities.
FAQs :
- What is a velocity model in seismic exploration?
- A velocity model describes how seismic waves travel through the Earth’s subsurface. It is essential for interpreting seismic data and identifying subsurface features such as oil, gas, and mineral deposits.
- Why are traditional methods of velocity model building challenging?
- Traditional methods are manual and iterative, requiring expert interpretation, which is time-consuming and prone to human error. These methods also struggle to scale effectively with the large datasets typical of modern seismic surveys.
- How does machine learning improve velocity model building?
- Machine learning enhances velocity model building by automating data processing, reducing human error, handling large datasets efficiently, and improving the accuracy of subsurface interpretations through advanced pattern recognition and predictive modeling.
- What types of machine learning are used in seismic data processing?
- The main types of machine learning used in seismic data processing are supervised learning (for predictive modeling), unsupervised learning (for clustering data based on similarities), and reinforcement learning (for optimizing decision-making processes).
- What is feature engineering in the context of seismic data?
- Feature engineering involves extracting and analyzing various attributes (such as frequency, phase, and amplitude) from seismic shot gathers to provide insights into the subsurface and aid machine learning models in distinguishing between different geological features.
- Can machine learning handle noisy seismic data?
- Yes, machine learning, especially deep learning techniques, can effectively manage noisy seismic data by identifying and separating important features from overlapping signals and intrinsic noise.
- What are the future prospects of machine learning in velocity model building?
- The future looks promising, with expected advancements in ML algorithms, integration with cloud computing, and improved data analytics enhancing the accuracy and efficiency of velocity models in seismic exploration.
- How have companies benefited from using machine learning for velocity model building?
- Companies in the oil and gas and mining industries have used machine learning to improve the accuracy of velocity models, leading to better drilling decisions, higher success rates in exploration, and reduced operational costs.
Read more information about Technology At trendaddictor